Analyzing Data Types: Understanding the Difference
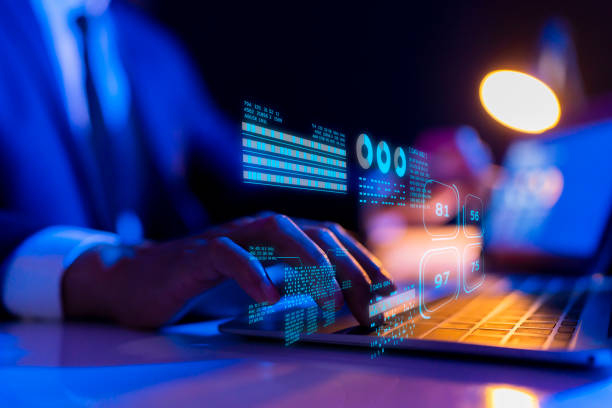
Introduction
In the world of data and statistics, the distinction between Nominal and Ordinal scales is fundamental. Even though some terms may sound alien to you, one you get the hang of the fundamentals, its pretty easy to navigate. Understand these scales and find out their significance in the field of research and data analysis.
Nominal vs Ordinal: Setting The Ground
Every research, survey, or data collection effort uses scales to categorise data. Among the most used are the Nominal and Ordinal scales. But what sets them apart?
Nominal Scale:
Definition: Nominal scales categorise data without giving any order or hierarchy. It’s all about labelling and naming.
Example: Think of colours – red, blue, and yellow. There’s no inherent order in these categories.
Ordinal Scale:
Definition: Ordinal scales don’t just categorise data; they also provide a sense of order. Here, categories have a rank.
Example: Imagine educational levels – high school, bachelor’s, master’s, and Ph.D. They follow a sequence from the least to the most education.
Applications in Real Life
You’d be surprised at how often we unknowingly use these scales in everyday decisions.
Nominal Data Applications:
Choosing a Flavour: When picking ice cream flavours like vanilla, chocolate, or strawberry, you’re dealing with nominal data.
Identifying Teams: In sports, team names represent nominal data.
Ordinal Data Applications:
Hotel Ratings: Hotels rated as 3-star, 4-star, or 5-star utilise the ordinal scale.
Customer Feedback: When customers rate services as poor, average, or excellent, they’re using an ordinal scale.
Advantages of Using Nominal and Ordinal Scales
Understanding these scales can optimise decision-making in various sectors.
Benefits of Nominal Scale:
Simplicity: No need for intricate calculations; just label or name.
Versatility: Suitable for a wide range of data types.
Benefits of Ordinal Scale:
Hierarchy Understanding: Gives a clear order or rank.
More Information: Provides a deeper insight compared to nominal data.
Statistical Measures Suitable for Each Scale
Choosing the right statistical measure is crucial. Let’s take a look:
Nominal Scale Measures:
Mode: The most occurring value.
Chi-square: Tests the relationship between two categorical variables.
Ordinal Scale Measures:
Median: The middle value.
Spearman Rank Correlation: Measures the strength and direction of the relationship between two ranked variables.
Common Misconceptions and Mistakes
Often, there’s confusion between these scales, leading to erroneous conclusions.
Nominal Misconceptions:
Nominal Data Can Be Ranked: Remember, nominal data is only about naming or labelling.
Ordinal Misconceptions:
Ordinal Data Intervals Are Consistent: The difference between ranks isn’t necessarily equal.
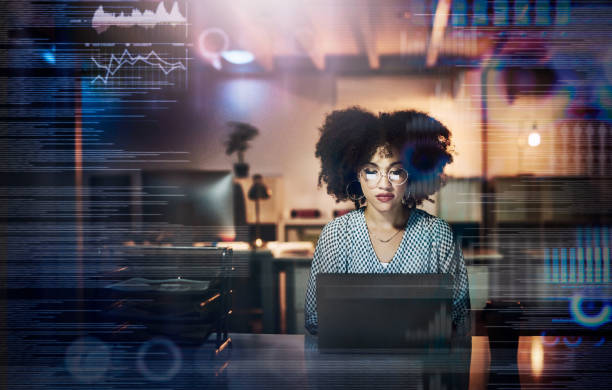
FAQs
- How do I determine if data should be nominal or ordinal?
Consider the nature of your categories. If they can be ranked or have an order, they’re ordinal. Otherwise, they’re nominal.
- Can ordinal data become nominal?
Absolutely! By removing the rank or order from ordinal data, it can be treated as nominal.
- Why can’t we just use one scale for everything?
Each scale has its unique strengths, and using the incorrect one can lead to misleading insights.
- Is age nominal or ordinal?
Age can be both. While the exact age is ratio data, categories like “young,” “middle-aged,” and “old” are ordinal.
- Can we use mean and standard deviation for ordinal data?
It’s controversial. While some statisticians argue it’s possible, many believe median is a better measure for ordinal data.
- Why is understanding these scales vital in research?
Scales determine the types of statistical tests to use, influencing the accuracy and reliability of results.
Conclusion
Understanding the distinctions between Nominal vs Ordinal data is crucial, not just in research but in everyday decisions. As you now grasp these differences and their importance, you can apply this knowledge in various realms, ensuring your decisions are data-driven and sound.
External Links/ Sources: