Explore the advantages of cluster sampling in research for accurate and efficient data collection
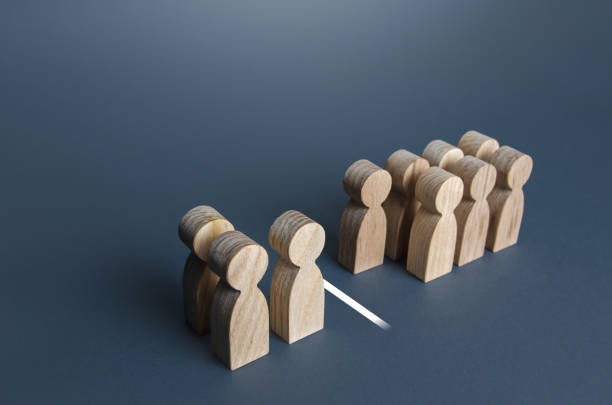
Introduction
In the evolving realm of research and data collection, one technique stands out like a beacon of hope: Cluster Sampling. For people unfamiliar with this, this approach offers streamlined data gathering while maintaining precision and efficiency. Before diving deep into its benefits, let’s unravel the layers behind this intricate methodology.
Cluster Sampling: The Heart of Efficient Data Collection
A cluster sampling approach involves the researcher dividing the whole population into sections and clusters, and opting for random samples from among the clusters. Each cluster represents the population, making it easier to conduct extensive surveys or experiments without having to reach out to every individual.
Origins and Evolution
Originating from the necessity to conduct large-scale surveys without incurring huge costs or time constraints, cluster sampling quickly became a preferred method among researchers. As the days progressed, research methods advanced and widened, the complexities of cluster sampling also grew, ensuring its position in the data collection history.
The Methodology Behind Cluster Sampling
This method differs from others in its approach. Here’s a step-by-step breakdown:
1. Divide the Population: The entire population is divided into clusters, often based on certain criteria or characteristics.
2. Random Selection: Random clusters are then chosen for further research.
3. Deep Dive: Every individual or element within the selected cluster is studied, ensuring a holistic approach.
Advantages Over Other Sampling Methods
What distinguishes cluster sampling from other methods is that it combines simplicity, efficiency and cost-effectiveness in a unique way. Even though some alternatives offer one or two of these advantages as well, cluster sampling is the one that delivers all three, making it the preferred option for most researchers.
Common Mistakes to Avoid
Like any approach, there are possible drawbacks to consider. Some typical errors involve the selection of clusters that don’t properly represent the data, a lack of understanding regarding the biases in the chosen clusters and failing to use proper techniques during analysis.
10 Miraculous Benefits of Cluster Sampling
1. Cost-Effective
By focusing on select clusters rather than the entire population, researchers can significantly cut down on expenses. Think about it: less travel, fewer resources, and minimised logistical challenges.
2. Time-Saving
Rather than trying to reach the entire population, researchers can focus on these clusters and thus, accelerate their process leading to faster outcomes.
3. Versatility
Regardless of the geographical boundaries, and the vastness of your population, cluster sampling easily accommodates a wide range of sizes and extents.
4. Greater Accessibility
In areas where the population is widely dispersed or hard-to-reach, selecting clusters makes the task feasible and less daunting.
5. Accuracy in Results
Accurate execution in cluster sampling method cane be as precise as when working with simple random sampling.
6. Flexibility in Analysis
Researchers can easily analyze both cluster and individual level data and enhance the comprehensiveness and depth of the findings.
7. Simplified Administration
Data management turns easier when originated from specific clusters as compared to random sources.
8. Enhanced Quality Control
With fewer clusters to monitor, maintaining the quality of data collection becomes more manageable.
9. Reduction in Non-Response Errors
When researchers focus on specific clusters, the chances of non-responses diminish, leading to more reliable data.
10. Suitable for Large Populations
In cases where the population size is massive, cluster sampling proves to be a pragmatic and efficient approach.
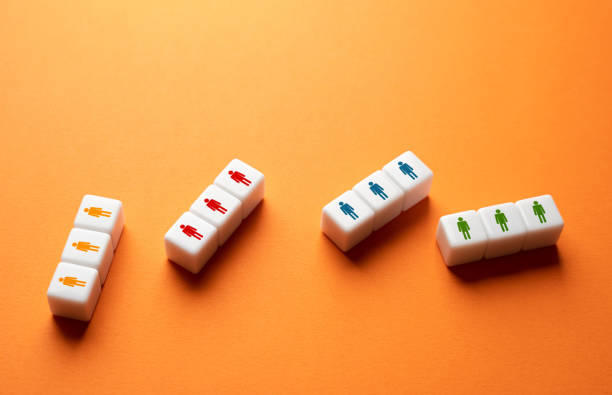
FAQs
- What is the primary purpose of cluster sampling?
Cluster sampling is designed to simplify data collection processes when studying large populations, making it both cost-effective and efficient.
- How is cluster sampling different from stratified sampling?
While both methods involve dividing the population, stratified sampling requires studying samples from all strata, whereas cluster sampling involves studying only the selected clusters in entirety.
- Is cluster sampling suitable for all types of research?
No, its effectiveness varies based on the research’s objectives and the inherent nature of the population being studied.
- How do you ensure the accuracy of results in cluster sampling?
By carefully selecting representative clusters and using correct statistical techniques during analysis.
- Can cluster sampling be biassed?
Yes, if non-representative clusters are chosen or if intrinsic biases within clusters are overlooked.
- Are there any significant drawbacks to cluster sampling?
One primary concern is the potential for higher sampling error if clusters are not chosen wisely or are inherently biassed.
Conclusion
Cluster Sampling, while intricate, offers a plethora of advantages that make it a standout method in the world of research. By understanding its nuances and leveraging its benefits, researchers can gather data more efficiently and effectively. Like any technique, being careful in approach along with combining a clear grasp on the basics ensures best results. While we strive to broaden our horizons of knowledge, its guaranteed that cluster sampling will be immensely significant in molding our comprehension of the world.
External Links/ Sources:
Cluster Sampling | A Simple Step-by-Step Guide with Examples