Benefits of Sampling in Research: Advantages Over Studying Entire Population
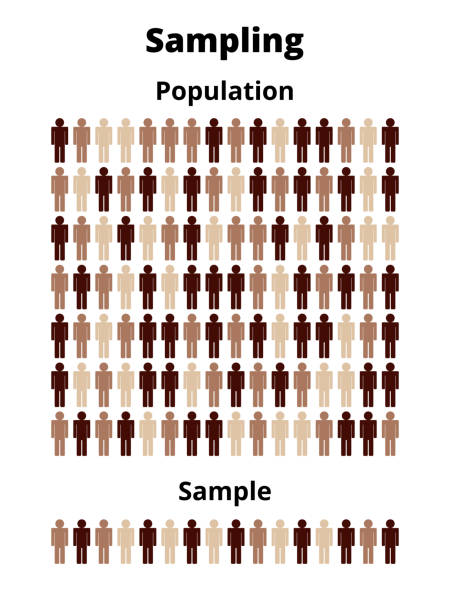
Introduction
Have you ever wondered why, when researching vast topics or studying intricate details, researchers opt for a sample instead of studying the entire population? Even if it seems counterintuitive initially, the reasoning behind the this is extensive and is rooted in practicality, efficiency and feasibility.
Why is a sample used more often than a population?
In the grand theatre of research, the sample plays a leading role. And for good reasons! A sample, when chosen correctly, mirrors the entire population’s characteristics without the hassles of surveying everyone. Here’s the inside scoop:
1. Cost-Effective: Gathering data from an entire population can burn a hole in the pocket. On the other hand, samples cut down costs significantly.
2. Time Saver: Imagine interviewing every individual in a country. It’s impractical, right? Samples save monumental amounts of time.
3. Accuracy Boost: Surprisingly, sometimes, a well-chosen sample can provide more accurate results than a disorganised attempt to study an entire population.
Diving Deeper: The Rationale Behind Sampling
The Science of Representativeness
To ensure a sample is effective, it should accurately represent the entire population, -much like how sampling a slice of cake gives you a sense of the whole dessert. If that tiny bite has all the flavors, you know exactly what the whole cake tastes like!
The Art of Randomization
In sampling, randomness is key. It ensures that every individual in a population has an equal chance of being chosen, making the results unbiased and genuine.
Feasibility and Accessibility
It is an insurmountable challenge to try and reach as entire population.
Think of studying deep-sea creatures or stars in a distant galaxy. Samples become the only logical choice.
Types of Sampling: Which One to Pick?
There are different ways to selecting a sample, and these methods are based on research’s characteristics, available resources, and the desired accuracy.
1. Simple Random Sampling: Everyone has an equal chance.
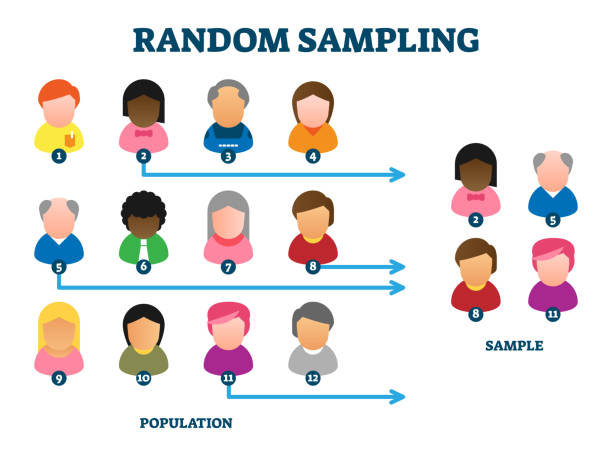
2. Stratified Sampling: Entails the segmentation of population into groups followed by the selection of samples from each of these groups.
3. Cluster Sampling: Utilizing geographical proximity for convenience, this method segments the population into clusters and selects a few to study them thoroughly.
4. Systematic Sampling: Choose every nth individual.
The Potential Pitfalls: Sampling Errors and Biases
Sampling isn’t foolproof. Like everything, it has its limitations:
– Sampling Error: The natural discrepancy that arises when a sample doesn’t perfectly reflect the population. A small sample leads to big potential errors.
– Bias: An uneven representation can skew results. It’s the arch-nemesis of randomization!
Samples in the Real World: Real-Life Applications
From political polling, product testing, to marketing surveys, samples are everywhere! Here are a few scenarios:
1. Medical Trials: Before a medicine hits the shelves, it’s tested on a sample.
2. Opinion Polls: Can’t ask everyone, so pollsters use samples to predict election results.
3. Quality Control: Manufacturers randomly test a few products from a batch, ensuring overall quality.
FAQs
- Why not just study the whole population?
Studying an entire population often isn’t feasible due to time, costs, and logistical constraints.
- Is sampling always the best choice?
Not always. For small populations or when high precision is needed, studying the entire group might be better.
- How big should a sample be?
It varies. The sample should be large enough to represent the population but manageable in terms of resources.
- Do all research methods involve sampling?
No, some qualitative methods, like case studies, focus on specific instances without generalising.
- What’s the difference between a sample and a census?
A sample studies a subset of the population, while a census attempts to include everyone.
- Can I trust the results of a sample?
If done correctly, yes. A well-chosen sample can accurately reflect the larger population.
Conclusion
“Why is a sample used more often than a population?” The answer lies in the intricate dance of feasibility, efficiency, and accuracy. Samples, when chosen with care, can mirror entire populations, offering insights that are both deep and wide.
External Links/ Sources: