Explore notable sampling error instances and their impact on research accuracy
Introduction
Sampling, a foundational concept in statistics, helps researchers draw conclusions about entire populations based on data from smaller groups. Errors always manage to happen, no matter the amount of planning undertaken. Let’s unravel the intricacies of sampling errors and illuminate them with examples for better understanding.
Sampling Error Defined
What is a Sampling Error?
In simple terms, a Sampling Error occurs when the statistics you got from the sample does not match those from the population. In essence, it’s the margin of “oops” we get when we don’t survey everyone in a population. If we were to measure and find the average height of 100 random people, the difference between the average height of those 100 people and the actual average height of the entire city is what we call Sampling Error.
Distinguishing Between Sampling and Non-Sampling Errors
While sampling errors emerge from the act of taking a sample, non-sampling errors have other culprits. These can arise from data entry mistakes, respondent bias, or even a faulty measuring instrument. Remember, a sampling error is purely about the difference between our sample and the true population, while non-sampling errors encompass a broader range of issues.
Exploring the Causes of Sampling Errors
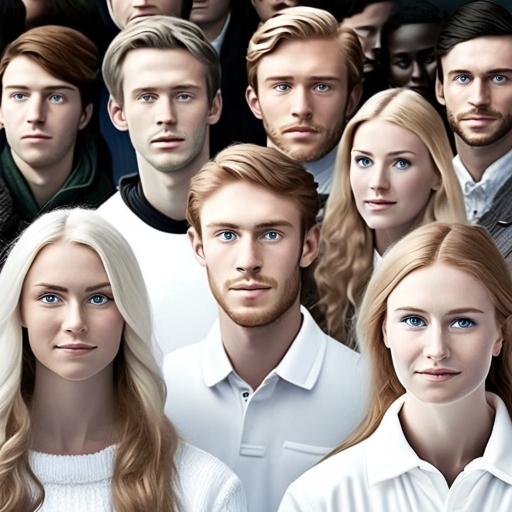
Inadequate Sample Size
Imagine trying to determine a nation’s favorite ice cream flavor by asking just five individuals. The chances of misrepresentation are sky-high! The chances of sampling error increase when the sample size is small. To get more precise results, it’s important to pick a sample size that truly represents all the different aspects of the whole group.
Non-random Sampling
Let’s say you’re researching urban gardening trends. If you only survey people at a gardening club meeting, you’re likely to get a skewed perspective. If the sample is selected in a biased manner, the likelihood of sampling error increases.
Population Variability
Some populations exhibit more variability than others. When you’re trying to find information about something that has a lot of different values or opinions, like political beliefs, there is a greater chance of sampling error when compared to studying something more consistent, like figuring out what candy a particular age group likes best.
Time-related Biases
Conducting a survey in college during the final exams week as opposed to at the beginning of the semester can lead to varied findings. Time, events, and seasons can impact people’s attitudes and responses, introducing a sampling error if not properly accounted for.
Sampling Error Example
Example 1: Biased Election Polling
We have witnessed it before, polls conducted before an election forecasts one result, but when the votes counted, the outcome is different. A classic sampling error example could be a poll conducted mostly in urban areas, ignoring the rural population’s preferences, leading to inaccurate predictions.
Example 2: Product Reviews and Sampling Errors
Companies often solicit reviews from their happiest customers, leading to an overly positive online image. But when new buyers don’t share that same level of satisfaction, we see a sampling error in action.
Example 3: Educational Surveys and Their Pitfalls
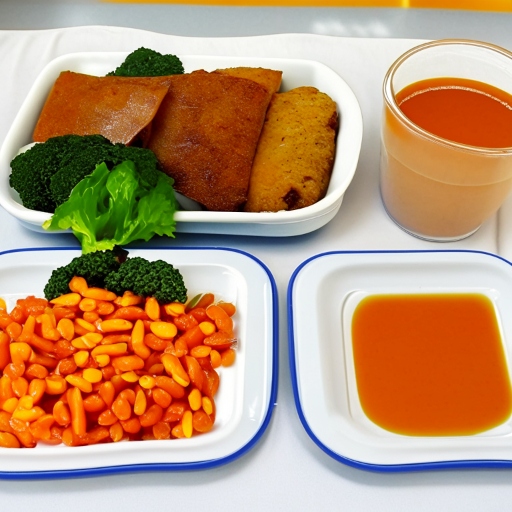
A school might survey students about cafeteria food during a special event with higher-quality meals. The overwhelmingly positive feedback would not represent sentiments about everyday meals, highlighting another sampling error.
Example 4: Market Research Missteps
A tech company releases a new app and gathers feedback only from tech enthusiasts. They miss the opinions of average users, leading to an app that’s too complicated for the general public. Once again, a sampling error skews perceptions.
Example 5: Medical Research Oversights
A drug trial might involve participants from a specific age group or health profile. When the drug is marketed to a broader audience without considering these differences, adverse side effects might emerge due to the sampling error.
Example 6: Environmental Data Sampling Slip-ups
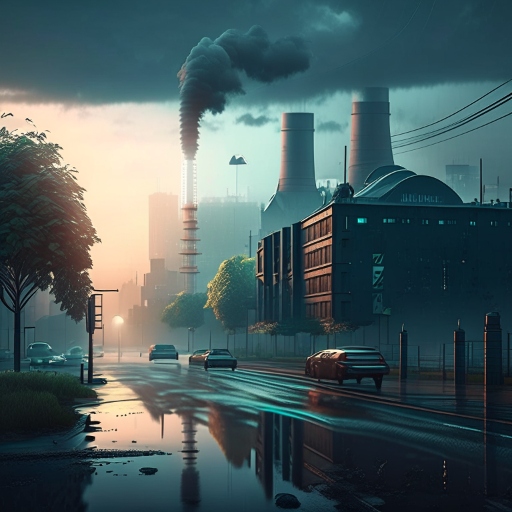
Research on city air quality taken on a day after heavy rain might show lower pollution levels than usual, giving a false impression of daily air quality.
Example 7: Social Media Trend Mistakes
Companies often monitor social media for trends. But if they only look for information from one place, say twitter, and don’t pay attention to other places like Facebook or Reddit, they could end up with an incomplete understanding, and come up with wrong strategies.
Mitigating Sampling Errors
Proper Sampling Techniques
Utilizing methods like Random Sampling can make sure that each person in a population has fair chance at being chosen, thus minimizing bias.
Increasing Sample Size
Using a bigger sample can give a better overall picture of the whole group, making it less likely that mistakes from smaller samples will have a big impact.
Utilizing Stratified Sampling
Dividing the population into subgroups and sampling from each can capture the diversity within the entire group, ensuring different segments are adequately represented.
Conclusion: The Ever-Present Need for Vigilance
In the intricate dance of statistics, sampling errors can trip up even the most astute researchers. By understanding their origins and being proactive in mitigating them, we can approach research findings with a more discerning eye. Rather than seeing a sampling error as a sign of flawed research, look at it as a call for you to study deeper, inquire more and strive towards a more accurate and factual data.
FAQs
- What is a sampling error?
A sampling error arises when statistics from a sample don’t align perfectly with those from the entire population.
- How can sampling errors be reduced?
Sampling errors can be minimised through proper sampling techniques, increasing sample size, and utilising stratified sampling.
- Are sampling errors a sign of bad research?
Not necessarily. Sampling errors are inherent in sample-based research. Recognizing and addressing them is crucial.
- Do larger sample sizes always mean fewer sampling errors?
Generally, larger sample sizes can reduce sampling errors, but it’s essential also to ensure randomness and representativeness.
- How do non-sampling errors differ from sampling errors?
While Sampling errors come from the act of sampling, non-sampling errors can arise from various other sources, like data entry mistakes or faulty measuring instruments.
- Is it possible to eliminate sampling errors entirely?
No, as long as we rely on samples instead of full populations, there will always be some degree of sampling error. The goal is to minimize and understand them.
External Links/Sources:
Sampling Errors in Statistics: Definition, Types, and Calculation