Exploring the Crucial Aspects of Correlation Matrices
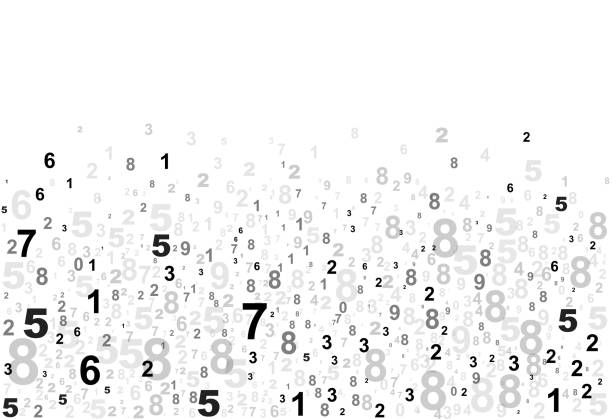
Introduction
The world is swamped with data. In this era of Big Data and advanced analytics, it is increasingly vital to extract valuable insights from this vast volume of numerical data.
Enter the Correlation Matrix. Rather than seeing them as number on a grid, look at them as a compass showing you through the maze of complex data relationships.
Correlation Matrix: The Navigator of Data Seas
At its core, a Correlation Matrix is a table showing correlation coefficients between variables. But why is it vital?
1. Revealing Hidden Patterns: With a Correlation Matrix, data patterns that aren’t immediately visible come to light.
2. Refining Predictive Models: If you’re into forecasting or predictive modelling, this matrix is your best friend.
3. Risk Management: In finance, it’s pivotal in portfolio management and understanding assets’ behaviour.
Decoding the Numbers
It’s not rocket science, but it does require a discerning eye. Each coefficient in the matrix denotes the relationship strength and direction between two variables.
– +1: Perfect Positive Correlation
– -1: Perfect Negative Correlation
– 0: No Correlation
And here’s a fun fact: ever heard the phrase “correlation doesn’t imply causation”? Well, it’s born out of this very matrix.
Tools to Create a Correlation Matrix
Thank heavens for technology! Tools like Python, R, and even Excel easily create a correlation matrix in seconds.
Significance Testing: Making Sense of It All
How do you know the correlation is not by mere chance? That’s where significance testing swoops in, ensuring the reliability of your matrix.
Applications in the Real World
You will be shocked by its versatility!
– Stock Market Analysis: Decoding market trends.
– Medical Research: Uncovering relationships between symptoms.
– Marketing: Identifying consumer behaviour patterns.
Pros and Cons: Every Rose has its Thorn
While the Correlation Matrix is a gem, it’s not without flaws.
Pros:
– Straightforward interpretation.
– Aids in hypothesis testing.
Cons:
– Might oversimplify relationships.
– Not always suitable for non-linear relationships.
The Future of Correlation Matrix
With AI and machine learning gaining traction, expect this matrix to evolve, becoming more refined and offering deeper insights.
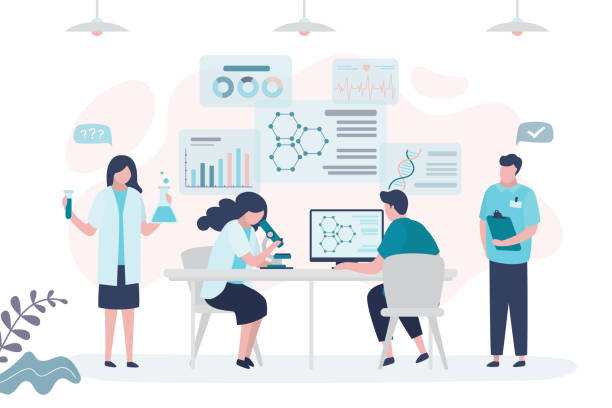
FAQs
- How is a Correlation Matrix different from a Covariance Matrix?
While both are tools in statistical analysis, the former focuses on the strength and direction of the linear relationship between two variables. In contrast, the latter measures the extent to which changes in one variable affect another.
- Why is the diagonal of the Correlation Matrix always 1?
Each variable’s correlation with itself is always perfect, hence the diagonal being filled with ones.
- Can a Correlation Matrix handle non-numerical data?
Typically, it’s designed for numerical data. However, with the right tools and techniques, one can compute correlations for categorical data too.
- What’s the difference between Pearson and Spearman in a Correlation Matrix?
Pearson measures linear relationships, while Spearman tackles monotonic relationships.
- Is a high correlation always good?
Not necessarily! It depends on the context. In some cases, high correlation might imply redundancy.
- Does a zero correlation mean no relationship?
No, it merely indicates no linear relationship. There might still be a non-linear relationship lurking.
Conclusion
The Correlation matrix acts as a beacon for researchers and analysist alike, and while it has its own challenges, its ability to demystify complex relationships is unmatched. So, when you are worried about navigating the sea of data, make sure to use this matrix as your navigation tool.
External Links/ Sources: