Unveiling the Complexities of Ordinal Data
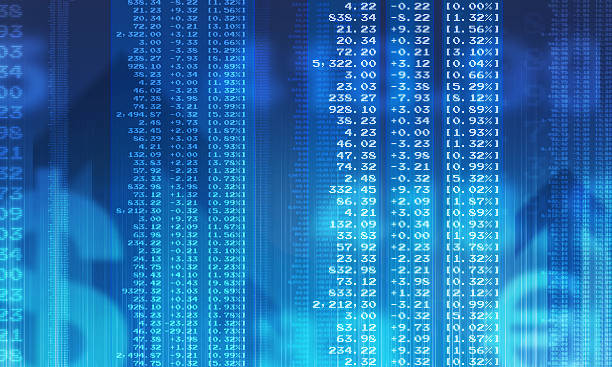
Introduction
Ever come across a ranking of your favorite movies, athletes, or food items? These rankings, though they may seem simple, are fundamental representations of what’s known as “Ordinal Data.” It’s not just numbers but numbers with a meaning, sequence, and an intrinsic order.
The importance of Ordinal Data can never be overstated in today’s age of data analytics and research, but what exactly is it? And what is its need? Read on to learn more about Ordinal Data.
Ordinal Data: The Basics
Understanding Ordinal Data is akin to understanding the building blocks of a ranked system.
What is Ordinal Data?
Ordinal Data is a type of categorical data with an order. Even though the categories have a sequences such as ‘first’, ‘second’ or ‘third’, the distance between them are unknown.
Characteristics of Ordinal Data
– Ordered Categories: The main feature of ordinal data.
– Undefined Distances: Distances between categories aren’t consistent or defined.
– Non-Numeric Nature: Even though numbers might represent them, these numbers lack a mathematical meaning.
Examples in Real Life
1. Socioeconomic status tiers like lower, middle, and upper class.
2. Rankings in a competition – gold, silver, bronze.
3. Ratings like poor, average, good, excellent.
Why is Ordinal Data Important?
In the vast universe of data, ordinal data holds a unique spot.
Role in Research
Ordinal Data aids researchers in gaining insights without needing exact numeric differences. Ordinal data is more detailed, and doesn’t delve into specifics unlike interval or ratio data.
Bridge between Categorical and Numerical Data
While providing order like numerical data, it also categorizes like categorical data, thus offering us the best of both.
Easy Collection and Interpretation
Both researchers and surveyors love working with ordinal data as its straightforward for respondents to provide and for the researchers themselves to analyze and interpret.
Collecting and Analysing Ordinal Data
Data is only as good as the insights drawn from it.
Methods of Collection
From surveys to observations, ordinal data collection is versatile.
– Likert Scales: Used in surveys, gauging agreement or satisfaction.
– Ranking Systems: Asking participants to rank items in a preferred sequence.
Analysis Techniques
Ordinal data might not have a mathematical meaning, but its analysis is vital.
– Median and Mode: Given the non-numeric nature, mean isn’t used.
– Non-parametric tests: Like Spearman’s rank correlation.
Pitfalls and Limitations
Like all data types, ordinal data isn’t without its shortcomings.
No Precise Differences
While the order is clear, how much better or worse one rank is from another isn’t defined.
Ambiguity in Interpretation
Especially in surveys, respondents might have personal scales, leading to ambiguity.
Not Suitable for All Analyses
Some mathematical operations and statistical tests aren’t valid for ordinal data.
Ordinal Data in Advanced Analytics
Ordinal data might seem simple, but its potential in advanced analytics is noteworthy.
Machine Learning and Ordinal Data
From prediction to classification, ordinal data has a role in the AI world.
Integration with Other Data Types
Ordinal data often complements other data types, like nominal or interval, to provide comprehensive insights.
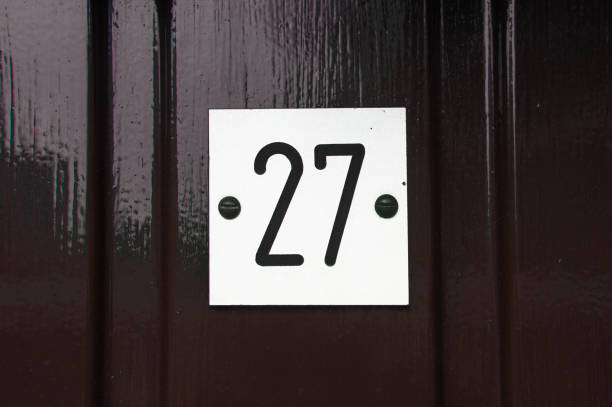
FAQs
- How is ordinal data different from nominal data?
Ordinal data has an order, while nominal data doesn’t. For example, ‘male’ and ‘female’ are nominal, but ‘low’, ‘medium’, and ‘high’ are ordinal.
- Can ordinal data be used in regression analysis?
Yes, but with caution. Techniques like ordinal logistic regression are designed for such data.
- What’s the main challenge with ordinal data?
The primary challenge is the undefined differences between categories, leading to potential misinterpretations.
- Is ordinal data qualitative or quantitative?
It’s qualitative as it deals with categories, but these categories have a specific order.
- How is ordinal data visualised?
Bar charts, pie charts, and stacked column charts are popular for visualising ordinal data.
- Can ordinal data be converted to interval data?
Not directly, since ordinal data lacks precise numeric differences. However, certain assumptions and techniques can approximate it to interval data.
Conclusion
Even though ordinal data is seemingly simple, it is vital for many research and analytical projects. It is both versatile and valuable as it provides us with the perfect balance between the clarity in categorical data and numerical data’s order. Understanding and learning about ordinal data becomes increasingly critical as data continues to drive decisions in the modern world.
External Links/ Sources:
What is Ordinal Data? Definition, Examples, Variables & Analysis