Discover the principles and applications of Correspondence Analysis in data visualization
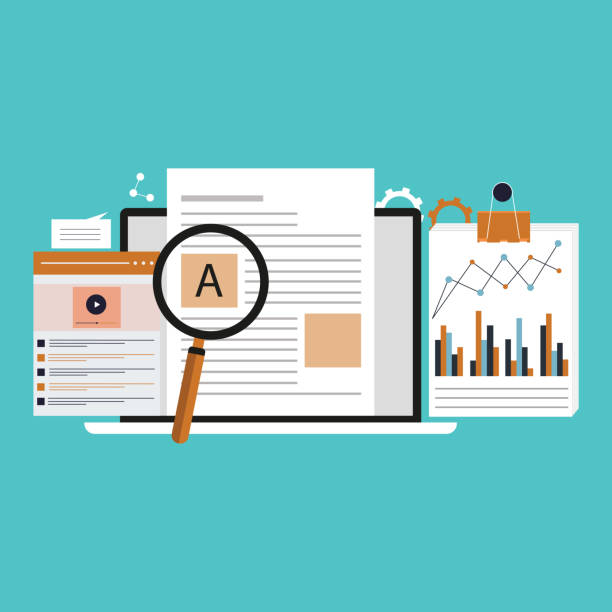
Introduction
In today’s data-driven world, analyzing and interpreting data is more crucial than ever. Among the myriad of analytical techniques available, correspondence analysis stands out for its utility and effectiveness. This guide will unravel the intricacies of correspondence analysis, shedding light on its applications, benefits, and how it has revolutionized the data analysis sector.
Correspondence Analysis: A First Look
Correspondence analysis, often referred to as CA, is a statistical technique used to visualise and interpret complex categorical data. At its core, CA seeks to identify and represent underlying patterns in a dataset. This technique is especially handy when dealing with large tables that can be challenging to interpret at a glance.
The Origins of Correspondence Analysis
Dating back to the early 20th century, CA has its roots in France, where it was initially developed as a method to analyse data tables. Its popularity has since grown, finding applications in various fields from marketing to ecology.
How Does It Work?
At its most basic level, CA transforms a data table into a visual representation, typically a map. Each point on the map corresponds to a category from the original table. The closer two points are on the map, the more similar their profiles in the data table.
Benefits of Using Correspondence Analysis
In the realm of data analysis, CA offers several advantages:
– Visualisation: Transforming complex tables into visual maps makes data interpretation more intuitive.
– Reduction of Dimensionality: CA condenses data, making it easier to identify patterns and relationships.
– Versatility: Suitable for various data types, from market research to biological data.
Practical Applications of Correspondence Analysis
As an analytical powerhouse, CA has seen diverse applications:
Market Research
Brands often use CA to understand consumer preferences and trends. By analysing purchase data, companies can gain insights into which products are popular among different consumer segments.
Ecology and Environmental Studies
Researchers use CA to study species distribution and environmental patterns. This helps in understanding ecosystems and formulating conservation strategies.
Linguistics
CA assists in analysing linguistic data, helping researchers understand language patterns, usage, and evolution.
Comparing Correspondence Analysis with Other Techniques
While CA is a robust tool, it’s essential to understand how it stands in comparison to other methods:
CA vs. Factor Analysis
While both techniques aim to reduce dimensionality, Factor Analysis primarily focuses on continuous variables, whereas CA deals with categorical data.
CA vs. Cluster Analysis
Cluster Analysis groups similar data points together, whereas CA provides a visual representation without necessarily clustering data.
Challenges and Limitations
Like all analytical methods, CA has its set of challenges:
– Interpreting Results: While CA provides a visual representation, interpreting these visuals can sometimes be challenging.
– Not Suitable for Small Datasets: CA works best with large datasets. With smaller datasets, the results might not be as reliable.
Future of Correspondence Analysis
The evolution of data science promises a bright future for CA. With advancements in technology, the application range of CA is only set to expand, making it an even more invaluable tool in the analytical arsenal.
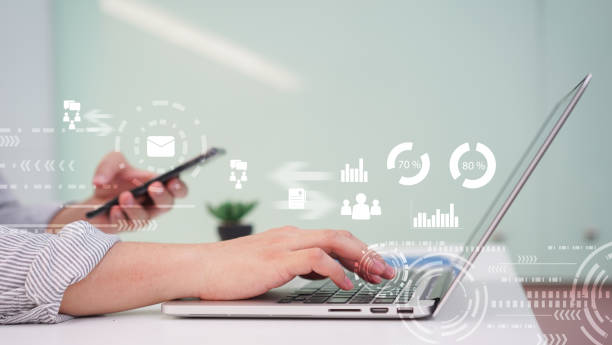
FAQs
- What is the primary purpose of correspondence analysis?
The primary purpose is to visually interpret and understand patterns in complex categorical data.
- How is correspondence analysis different from other analytical methods?
CA focuses on visual representation and is specifically tailored for categorical data, making it unique from other techniques.
- Is correspondence analysis suitable for all types of data?
No, CA is best suited for large datasets, especially those containing categorical data.
- Are there any drawbacks to using correspondence analysis?
Yes, interpreting the visual results can sometimes be challenging, and it might not be ideal for smaller datasets.
- Which industries primarily use correspondence analysis?
It’s widely used across various sectors, including market research, ecology, and linguistics.
- How has technology impacted the evolution of correspondence analysis?
With advancements in data science and technology, the application range and efficiency of CA have significantly improved.
Conclusion
Correspondence analysis is undoubtedly a game-changer in the world of data interpretation. Its ability to transform complex data into visual insights makes it a preferred choice for analysts and researchers. Whether you’re in market research, ecology, or linguistics, understanding and leveraging CA can give you a competitive edge.
External Links/ Sources: