Introduction to Non-Response Bias
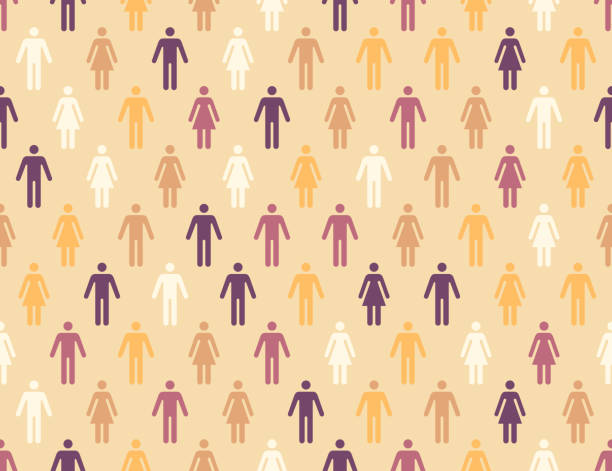
The term Non-Response Bias might sound intricate, but it’s a common phenomenon in research, surveying, and polling. This bias arises when certain individuals or groups do not respond to a survey, leading to a skewed representation of the larger population. The roots of this bias trace back to the earliest days of research, where varying response rates led to skewed results.
Definition and Basics
At its core, Non-Response Bias occurs when the responses collected in a survey do not represent the intended population accurately. It’s like trying to paint a portrait without all the necessary colors—what you end up with isn’t a true representation.
Historical Overview
The history of Non-Response Bias is as old as surveying itself. From the ancient census takers of Rome to today’s online surveys, researchers have always grappled with the challenge of ensuring complete representation.
The Importance of Addressing it
It might seem trivial, but unchecked Non-Response Bias can wreak havoc on research conclusions.
The Impact on Research Results
Imagine a scenario where only the satisfied customers of a product review it online. The feedback might be overwhelmingly positive, but it doesn’t capture the entire sentiment. That’s the danger of Non-Response Bias in a nutshell.
Misleading Interpretations
This bias can lead to flawed interpretations. For instance, a survey aiming to gauge employee satisfaction might misrepresent if disengaged employees choose not to respond.
Types of Non-Response Bias
Just like a coin has two sides, there are distinct types of Non-Response Biases.
Unit Non-Response
Here, entire units (like households or individuals) do not respond to a survey. It’s the equivalent of missing puzzle pieces.
Item Non-Response
In this type, respondents might skip specific questions, creating gaps in data.
Post-survey Adjustments
Sometimes, researchers adjust data post-survey to account for non-responses. However, these can lead to newer biases.
Non-Response Bias
In any research or study, ensuring accurate, unbiased results is of paramount importance. However, Non-Response Bias acts as a silent disruptor. It’s like a wolf in sheep’s clothing—often unnoticed but significantly impactful.
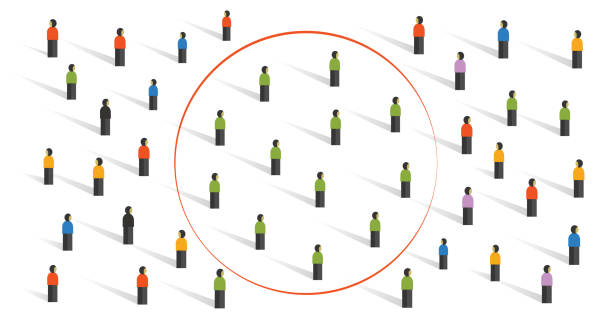
FAQs
– What is the main reason behind Non-Response Bias?
The primary reason is the differential rates of responses among various subgroups within a sampled population. This can arise from various factors, including survey design, medium, or even the topic itself.
– How does Non-Response Bias differ from Response Bias?
While both pertain to biases in survey responses, Response Bias deals with inaccuracies from respondents’ tendencies, while Non-Response Bias arises from the absence of responses from certain segments.
– Are there modern tools to combat this bias?
Absolutely! With the rise of technology, tools like AI and machine learning algorithms can predict and counteract potential biases, ensuring more accurate research results.
– Does online surveying reduce Non-Response Bias?
Not necessarily. While online surveys make participation easier, they come with their set of challenges, like digital accessibility and engagement issues.
– What industries are most affected by Non-Response Bias?
Any sector relying on surveys or research can be affected. This includes market research, healthcare, social sciences, and even political polling.
– Can Non-Response Bias ever be fully eliminated?
While it’s challenging to eliminate entirely, with careful planning, thoughtful design, and the right tools, its impact can be minimized significantly.
Conclusion
The journey through the intricacies of Non-Response Bias offers profound insights. The bias, while omnipresent, is not undefeatable. With the right knowledge, tools, and strategies, researchers can ensure that their findings are not just numbers but accurate representations of reality. As we move into an era of big data and advanced analytics, addressing biases like these will be more crucial than ever. After all, in the search for truth, every response counts.
External Links/ Sources: